How AI Helps Ensure Compliance and Integrity Monitoring in Private Companies
admin • Aug 19,2024
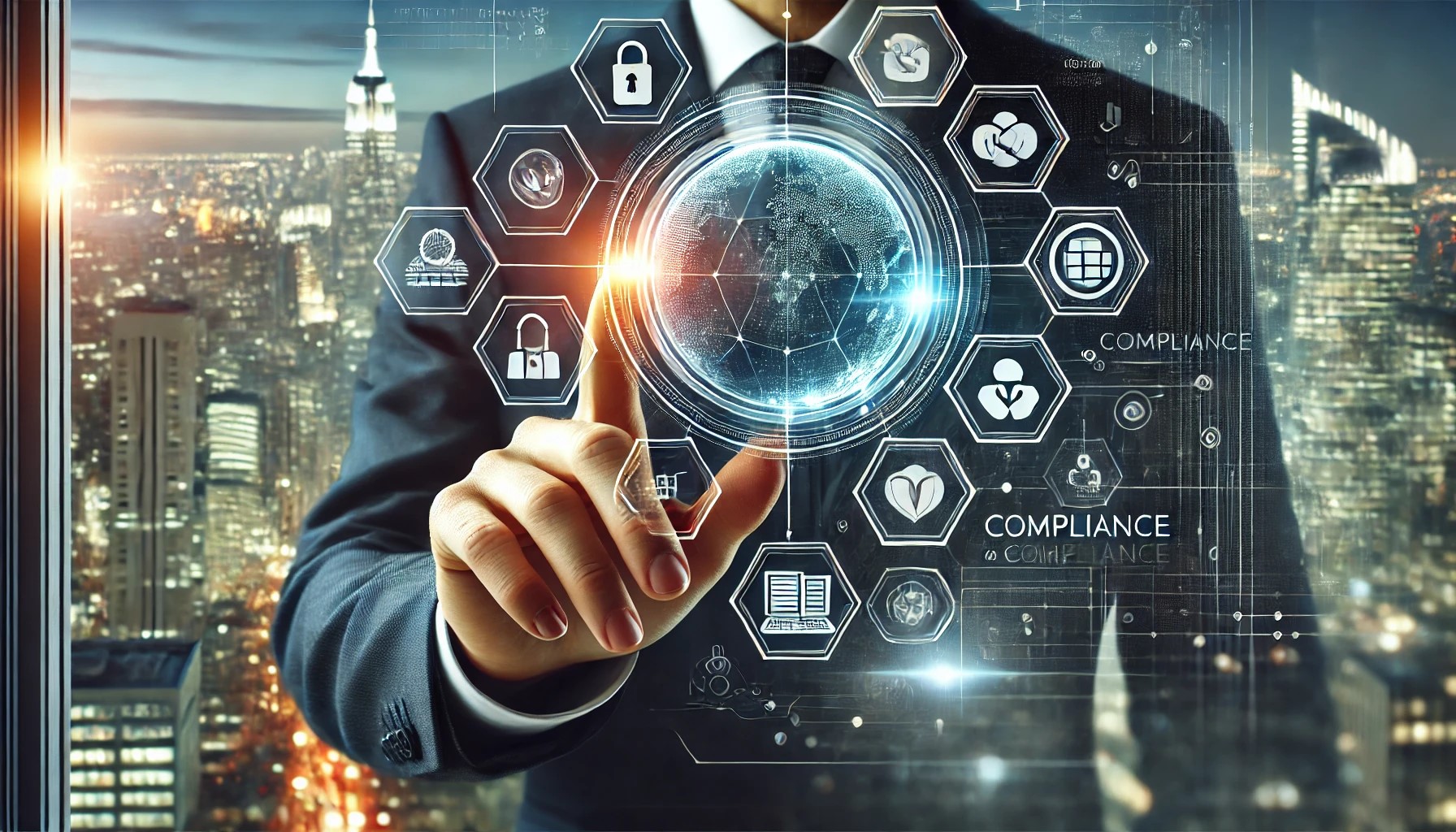
In today’s world, where regulatory requirements and ethical standards are becoming increasingly complex and stringent, private companies face numerous challenges in ensuring compliance and integrity monitoring. Failure to meet these requirements can result in significant financial penalties, legal sanctions, and the loss of trust from customers and partners. In response to these challenges, artificial intelligence (AI) is emerging as an indispensable tool for maintaining transparency, legal compliance, and ethical standards in business.
AI offers a range of innovative solutions that enable companies to effectively and automatically monitor compliance with regulatory requirements, detect potential risks, and ensure a high level of integrity within organizations. Machine learning algorithms and data analytics can process vast amounts of information, analyze transactions, identify suspicious patterns, and predict potential violations before they occur. This allows companies to act proactively, preventing issues rather than responding to them after the fact.
Moreover, AI helps automate routine compliance processes such as document verification, reporting, and auditing, which significantly reduces the risk of human error and increases efficiency. Modern AI-based solutions can also include interactive platforms for feedback and communication monitoring, fostering a more integrated and informed corporate culture within companies.
AI can significantly aid in compliance and integrity monitoring through several key methods:
- Data Analysis: AI can process large volumes of data to detect anomalies or inconsistencies that may indicate violations of norms or standards.
Examples of using AI for Data Analysis:
1. HSBC collaborated with Ayasdi, a company that develops machine learning (ML) software, to create an AI-based solution for combating money laundering. This software is designed to detect suspicious patterns in historical data related to money laundering. By analyzing current payment data, it quickly identifies fraudulent patterns in transactions and alerts personnel to block such payments. The software examines various factors, including the source and destination of payments, to identify any unusual behavior. By implementing this AI-based anti-money laundering (AML) solution, the bank was able to reduce investigation time by more than 20% and minimize regulatory risks by identifying risk segments that were previously overlooked.
2. Similarly, JPMorgan Chase implemented an AI-based system to enhance its AML efforts. This system uses ML algorithms to analyze customer data and identify potential risks. Integrating AI-based solutions into compliance processes for combating money laundering allowed the bank to detect fraudulent documents by analyzing trends and violations, significantly improving the ability to identify fraudulent activities. The company achieved better accuracy and faced a 95% reduction in false positives.
3. The Valley Bank AML team sought to simplify the process of detecting money laundering activities across millions of transactions by reducing the amount of manual work required for predictive modeling. Working with data processing specialists from DataRobot, Valley Bank developed and validated over 100 models for more accurate identification of likely money laundering transactions. The technology tests and deploys these models with minimal effort required from Valley Bank personnel. The AI-powered cloud solution for banking includes automated modeling for the bank’s AML team, resulting in a 22% reduction in false positives and a three-point increase in the escalation of money laundering alerts.
- Process Automation: AI automates routine tasks such as regulatory compliance checks or document updates, reducing human error and enabling rapid monitoring.
Example of using AI for Process Automation:
- The Intelligent Passenger Registration System at Daxing Airport automatically verifies passengers’ identity documents and flight tickets before departure. The ML model underlying the software for this system was trained on thousands of images and videos from common open-source libraries, including cloud services, social networks, mobile device uploads, and embedded hardware platforms. The AI algorithm can identify and detect potentially anomalous images or frames. The system alerts staff in real-time about these anomalies for further inspection. By using AI technology for facial recognition, the airport has significantly improved operational efficiency and passenger satisfaction.
3. Risk Prediction: AI can forecast potential risks based on historical data and behavioral patterns, allowing companies to proactively take measures to prevent violations.
Examples of using AI for Risk Prediction:
1. LifeScan, a leading global medical device company specializing in blood glucose monitoring, aimed to implement a software suite to mitigate and detect risks related to fraud, corruption, sanctions, kickbacks, and conflicts of interest. Lextegrity’s software AI solution provided LifeScan with a comprehensive overview of transactional expense and revenue risks within the organization. This AI solution enhanced the company’s compliance program within 90 days, offering automated screening for sanctions, watchlists, and adverse media checks on fund recipients, with the ability for users to process potential matches directly within the application.
2. The world’s largest brewer, AB-InBev, developed an ML technology to identify risky business partners and potential illegal payments. The BrewRIGHT analytical platform was created to consolidate data from finance, compliance, HR, and other systems, improving the detection of transactions and third parties that pose risks. By gathering data from operations in over 50 countries, BrewRIGHT enables proactive monitoring of legal risks and prevents violations. The AI platform is built on a risk assessment approach, where transactions or relationships are classified as high-risk based on various risk attributes (such as urgency of payment, payment to a political or government entity, and supplier type) and a weighted scoring of these attributes. This AI-driven solution reduced the company’s costs related to compliance with anti-corruption norms by millions of dollars.
4. Document and Report Analysis: AI can rapidly review and analyze legal and compliance documents, identifying potential issues or inconsistencies.
Examples of using AI for Document and Report Analysis:
1. A European distributor of agricultural machinery initiated an internal investigation into cartel activity. Management needed to respond quickly to mitigate the risk of fines and negative publicity. The primary objectives were to determine whether the bid-rigging was an isolated incident involving a single fraudulent employee or a more widespread issue. The company employed the Aiscension tool, developed in collaboration with eDiscovery and AI experts at Reveal Brainspace, designed to rapidly scan millions of messages using AI, providing exceptional speed and efficiency in compliance monitoring. This tool allows for proactive and swift scanning of communications for potential risks. Working in over 100 languages, Aiscension can be implemented across the entire supply chain to ensure compliance. Aiscension is trained to detect various forms of cartel behavior, including price-fixing, bid-rigging, market division, collective boycotts, and the exchange of confidential information. The Aiscension tool enables investigations or compliance checks to be conducted 91% faster than traditional audits and more cost-effectively than any other options.
2. The Microsoft Compliance Analytics Program Solution for real-time compliance monitoring throughout the lifecycle of a trading agreement utilizes advanced digital technologies, including RPA/IPA, ML, AI, and data visualization, to detect corruption risks at the transaction level. This specialized solution has integrated enhanced controls into Microsoft’s standard sales process. Microsoft pre-assigns risk scores to its sales contracts and trading partners on a scale from zero to 100. When a contract or partner exceeds a certain risk threshold or score, a report is generated for the compliance function embedded in the business to exercise oversight. By identifying corruption risks early in the sales lifecycle, Microsoft has been able to effectively mitigate risks and assess the adequacy of existing controls.
5. Communication Monitoring: AI can scan emails, messages, and other communications to detect signs of corruption or policy violations.
Example of using AI for Communication Monitoring:
- Canadian digital finance company Mogo, which provides digital solutions to empower consumers in managing and overseeing their finances, faced challenges in properly identifying politically exposed persons (PEPs). Mogo did not want to rely on outdated lists of public figures, which made the identification process time-consuming and unreliable. To address these issues, Mogo decided to transition to an AI-powered screening and object recognition platform. The company’s integration of an AI-driven AML platform with deep learning models and neural networks enabled compliance teams to identify PEPs 300 times faster, leading to a 55% reduction in investigation costs.
Conclusion
The implementation of AI in compliance and integrity monitoring opens new horizons for private companies, ensuring higher levels of security, transparency, and accountability. This not only reduces risks but also strengthens brand trust, enhances reputation, and improves competitiveness in the market. In the future, the role of AI in these processes will only grow, unlocking new possibilities for maintaining high standards of integrity and ethics in business.