ML gives a comprehensive picture of city traffic
A I • Jun 30,2024
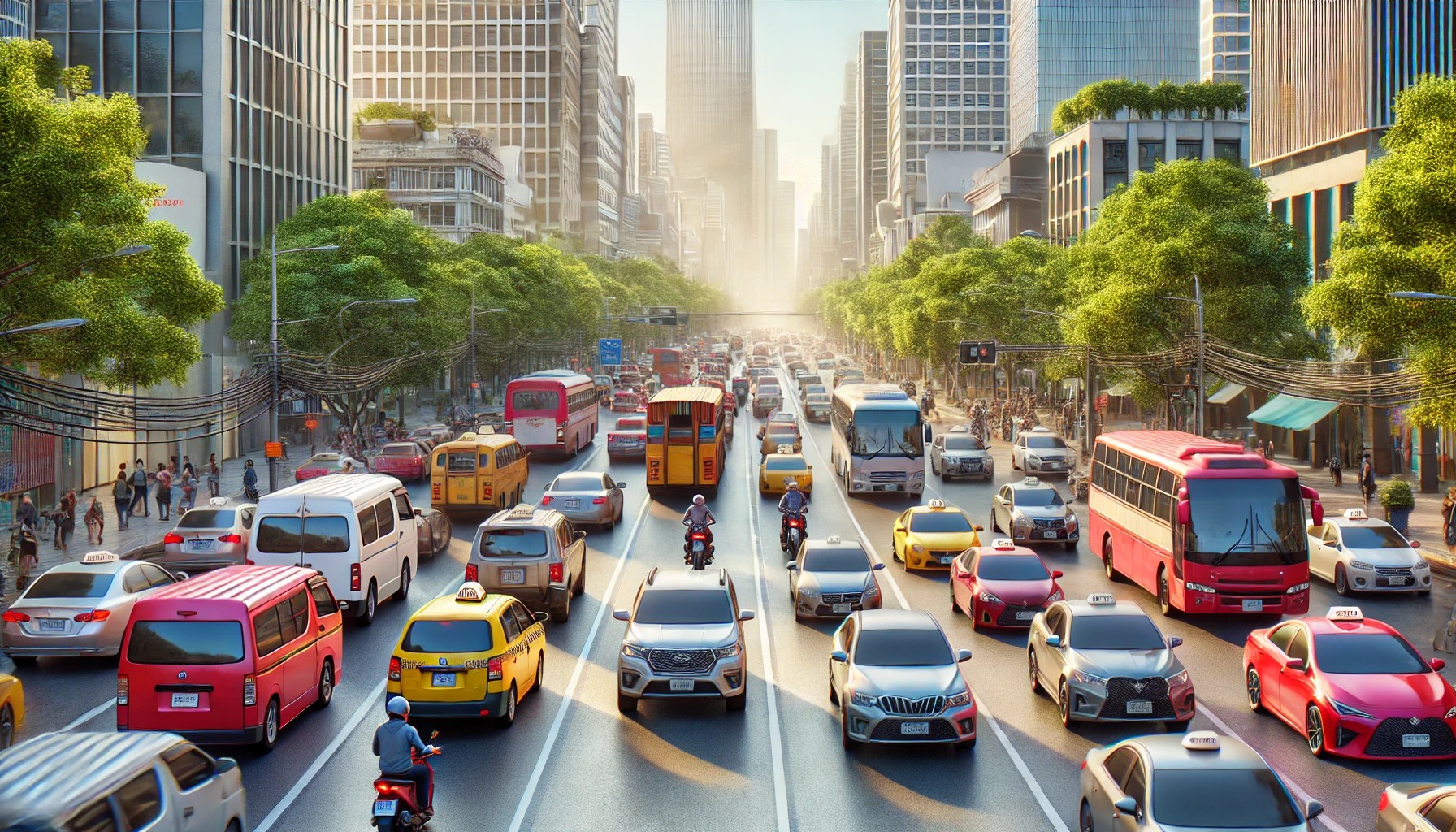
Summary:
The ML algorithm is designed to assist urban transportation analysts by providing practical insights into city traffic patterns. It can make nearly real-time estimations at the street level.
Client:
The U.S. Department of Energy
Problem Statement:
Traffic engineers need dependable data to assess congestion in transportation networks, identify bottlenecks, and guide their planning and response strategies. However, available traffic data is often limited, incomplete, and mostly historical, focusing on collision reports and isolated traffic counts.
Traditionally, engineers have used these limited datasets to evaluate road conditions. There was a need for a new tool to provide urban traffic engineers with actionable insights into city traffic patterns.
Results:
- A reduction of the time needed to develop a traffic congestion model by 10 times from days down to a few minutes by integrating TranSEC with public data across the 1,200-square-mile Los Angeles metropolitan area.
- Near-real-time traffic analysis feasible.
- Improved situational awareness within transportation management centers.
AI Solution Overview:
A ML algorithm called TranSEC (Transportation State Estimation Capability) has been developed at the Pacific Northwest National Laboratory (PNNL) under the U.S. Department of Energy to tackle urban traffic congestion. It aims to provide urban traffic engineers with actionable insights into city traffic patterns.
TranSEC stands out from traditional traffic monitoring methods by effectively analyzing sparse and incomplete data using ML. This capability allows it to fill gaps left by older data collection methods and make near real-time street-level traffic estimations.
The tool utilizes traffic data from UBER drivers and other publicly accessible traffic sensors to map street-level flow over time.
By leveraging ML and the computing power of a national lab, it creates a comprehensive view of city traffic. Unlike smartphone navigation apps that assist individual drivers, TranSEC is designed to help reduce congestion for all vehicles, enhancing overall traffic efficiency.
As more data is collected and processed, the ML component of TranSEC improves, making the tool more accurate and beneficial over time. It employs graph-based models, innovative sampling techniques, and optimization engines to provide insights into travel times and routes.
References:
- A New ML Algorithm To Manage Traffic Congestion In Cities. https://indiaai.gov.in/news/a-new-ml-algorithm-to-manage-traffic-congestion-in-cities
- New Machine Learning Tool Tracks Urban Traffic Congestion. https://www.eurekalert.org/news-releases/882754#:~:text=The%20tool%2C%20called%20TranSEC%2C%20was,traffic%20patterns%20in%20their%20citie
- Transec: Tracking And Alleviating Urban Congestion. https://www.pnnl.gov/sites/default/files/media/file/DDST_0134_FLYER_TranSEC_WEB_V2_0.pdf
- TranSEC: Tracking and Alleviating Urban Congestion. https://www.pnnl.gov/available-technologies/transec-tracking-and-alleviating-urban-congestion
Industry: Public Services
Vendor: Pacific Northwest National Laboratory
Client/Clients: The U.S. Department of Energy
Publication Date: 2020